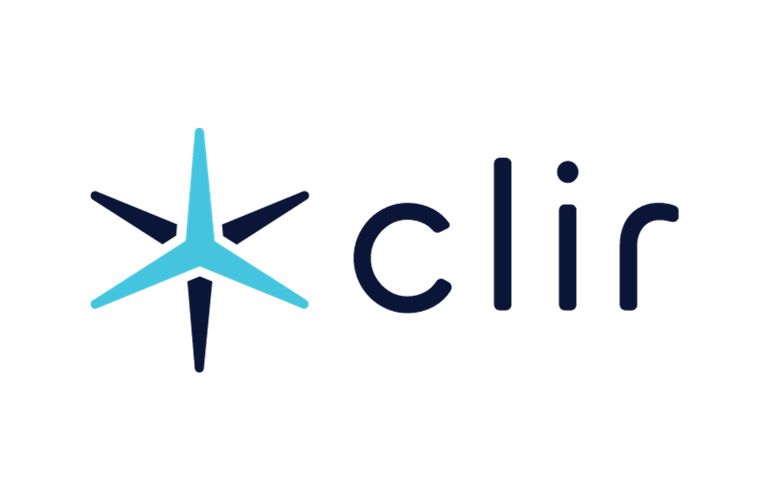
Clir Renewables has released new technology to model the variability of hourly energy production. Powered by machine learning and over 200 GW of data, the software greatly improves upon the accuracy of industry standard approaches to modeling production shape and volume. The result is quality data to support owners and operators with production and revenue forecasting to optimize offtake strategy.
Beyond fifteen days, weather forecasts are not useful for renewable energy production forecasting, so owners rely on statistical models. These models vary in sophistication, but often do not properly account for the hourly variation in generation at the site or for the actual production history of operating projects.
Clir’s data scientists have overcome many of the pitfalls of standard statistical models. A machine learning model is trained using hourly gross energy from the wind or solar farm and climate data from the corresponding period. The projections from the trained model are then tested against actual production in the years following the training data period. Once trained, the model is used to produce a time-series distribution based on more than twenty years of data. The result is a simulation of hourly gross energy production uncertainty bands in any month, quarter or year based on the historical trend.
Once the gross hourly production is generated, Clir uses intelligence from over 200 GW of operational assets to account for the expected energy losses of the farm. The validated gross model is trained on peer loss data — based on technology type, asset age, location, size, environmental conditions and other factors — to simulate the net energy production uncertainty bands in any hour, day, month, quarter or year.
One of the key applications for the new volume modeling platform is power production hedges. Hedge contracts are commonly used to provide revenue certainty in markets where owners are exposed to fluctuating market prices. Regardless of whether these hedge contracts settle hourly or less frequently, having an accurate view on the range of likely energy production outcomes can help ensure the contracts are optimal. By looking at the distribution of expected production across several assets, and accounting for the impact of diversification, portfolio-wide hedges can also be optimized.
“Our new production volume modeling platform will allow renewable energy infrastructure investors and operators to realize millions in revenue potential,” said Gareth Brown, CEO of Clir Renewables. “We recently teamed up with two major wind investors to test the models on over $2 billion dollars of assets and, in both cases, there was a substantial difference in their production expectations based on previous assumptions. The benefits to the customer are millions of dollars in gains to both today’s assets and the future revenue streams of the assets.”
News item from Clir Renewables